The intent of this discussion is to illustrate the impact that IIoT when combined with Artificial Intelligence (AI) can have on achieving a state of true predictive awareness and management of the health of industrial assets. This approach is often referred to in the industry as predictive maintenance.
Although partially true, the term predictive maintenance understates the potential of AI and IIoT; which is a cognitive industrial asset.
A cognitive industrial asset is always aware of its health – measured by the asset’s ability to perform its intended function – and able to provide a warning about the state of its health and the causes of the problems.
For the sake of consistency with industry convention, we’ll continue to use the term predictive maintenance.
The intent of this discussion is not to describe the value and virtues of moving from reactive or time-based maintenance to predictive maintenance, but to provide information on the application of IIoT and AI (particularly machine learning) to achieve true, valuable predictive maintenance.
Condition Based Maintenance
Condition Base Maintenance (CBM), a significant improvement over time-based maintenance, is what is often referred to as predictive maintenance. CBM is characterized by the use of measurement of symptoms of the degree of degradation of the health of an asset. The symptoms are referred to as conditions.
The very premise of CBM is that the asset’s health has degraded to a point that it can be detected by measuring a physically detectable condition. On the classic P-F curve, this point is referred to as the potential failure point, “P”.
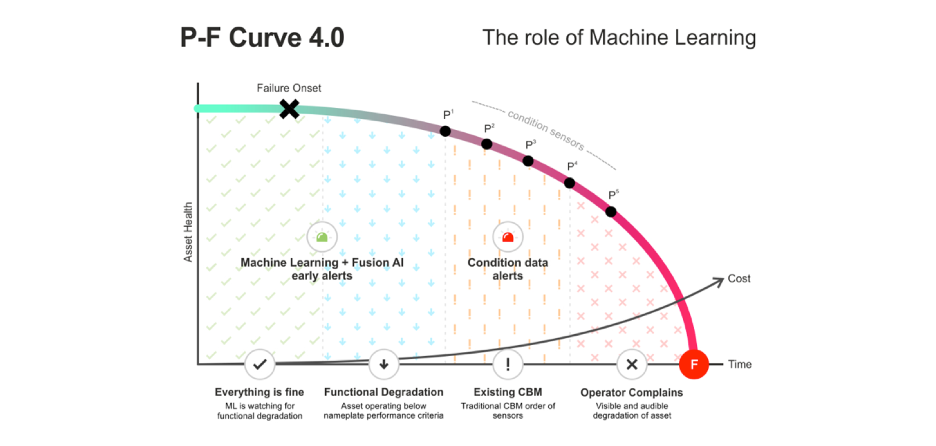
Typical condition measurements include temperature, vibration, acoustics, motor current, oil tribology, etc. The key objectives of CBM are to detect these conditions early; be able to determine severity, the cause or failure mechanism; and assess the time remaining when the asset’s health will degrade to an intolerable level.
Depending on the condition measurement technique used, asset health degradation can be detected earlier (or farther up the P-F curve). For example, it is believed that ultrasonic sensors can detect rotating (or moving) asset problems the earliest (indicated by P1).
Insights and outcomes are typically achieved through analysis of the condition data as follows:
- Condition data is analyzed by human machinery or asset health analysts. Signal processing and visualization techniques have continued to improve to make this analysis easier, but the quality of the outcomes depends on the experience of the analyst and their intimate knowledge and understanding of the asset’s operation.
- The analyst will typically define “baseline” values for one or more condition variables and observe the trend of divergence from the baseline to assess the degree to which asset health has degraded. Often, the analyst will define multiple baselines for the different operating modes or states of the asset’s operation.
- An improved technique in online systems allows for alarms and alerts for divergence from baseline can be set and “triggers” based on asset operation state are set to capture more granular data.
While CBM offers a significant advantage over time-based maintenance, it has the following limitations and challenges for widespread adoption and usefulness:
- The need for human analysis requiring a high degree of expertise and understanding can be a limitation when monitoring several assets.
- The operational context impacts the definition of baseline or “normal” condition values. True values from a condition measurement can only be obtained by reviewing them in the context of the operation such as load, process fluid conditions, steady state, and transient operating states. For industries where assets’ operating modes need to vary a lot, it becomes increasingly difficult, and sometimes, impossible (such as complex batch operations) for human analysis to assess normal and abnormal condition levels.
- The baselines can change over the lifecycle of the asset.
- The rule-based alarms and triggers defined for online systems can become static and must be maintained and changed to remain relevant.
- Combining assessment from more than one condition variable allows for a higher degree of accuracy and certainty about the asset’s health. This is difficult to achieve through human analysis, and online condition monitoring systems rarely allow for such fusion of data due to the limitations of rule-based logic that must be programmed.
Using Machine Learning to build predictive maintenance with CBM
Machine Learning can be used to overcome all the challenges and shortcomings of traditional CBM. If you have already invested in CBM technology, you can quickly increase the ROI and usefulness of your investment by using the machine learning capabilities of the Quartic Platform™.
If you are evaluating or implementing a new CBM system, taking a modern approach with IIOT and AI will help you achieve higher and faster ROI.
1. Make condition monitoring dynamic and automate the analytics to generate insights by combining process and operation context with condition data.
2. The definition of baselines and “triggers” becomes infinite with Machine Learning. The Machine Learning algorithm learns the “normal” patterns (not just amplitude values) of any condition variable (such as vibration) and provides dynamic detection of “abnormal”
3. A number of equipment health problems develop during transient states (such as start-up, ramp-up, raw material grade change, etc.). The machine learning algorithm monitors not just the steady state, but also the normal and abnormal patterns for transient states. Conditions that are only observable under a transient state are therefore continuously monitored.
4. Most equipment health degradation is gradual. Simple alert thresholds on condition variables either limit the early warning or result in too many spurious alerts. By applying rules to the output of the machine learning agents, you can create many early warnings and avoid nuisance alerts.
5. With the rules engine and complex event processing (CEP) included in the Quartic Platform™, you can combine multiple condition variables (such as temperature and vibration) in the operational context to further increase the accuracy and trust in the equipment health predictions.
6. Machine Learning agent output can also be combined with the rules engine (Reckon) to build a root cause indicator.
Note: Machine learning is not a magic box. If you do not understand the operation, failure modes, and mechanisms of the asset, it will be difficult to achieve this ROI.
But, if you can bring together your subject matter experts to design and define the problem you are trying to solve, you can build the machine learning models yourself with Quartic eXponence™ – without any data science or machine learning, or programming knowledge.
The true value of AI – early detection of asset health degradation
As noted in the CBM description, asset health prediction can only be made once the health has degraded to the potential failure point “P”. It is understood that in this stage the asset is performing its intended function in a reduced capacity and eventual failure is impending.
The fact is that before the asset reaches this stage, it goes through a phase of process degradation – in this stage, the asset is performing its intended function but in a diminished capacity.
The degradation of performance is below the nameplate expectations but not bad enough to cause disruption of the process.
The asset may be “working harder”, consuming more energy, or introducing more variability but operations are able to overcome this degradation – they don’t yet feel the need to “call maintenance”.
Such degradation is typically operations or process-induced degradation and if not corrected will eventually lead to the onset of “P”.
This area of degradation is a real opportunity for true predictive asset health monitoring. While most of the signals needed to detect this state are available within traditional process control systems used to operate the asset, it is not possible to implement predictive health monitoring with these traditional logic-based systems.
This is the ideal implementation of machine learning. In this approach, key indicators of process degradation are identified and machine learning models (or agents) are developed to learn the “normal” or baseline behavior of these indicators.
Gradual, sustained divergence trends from the normal are used to provide an early indication of degradation. Additionally, key features, or influencing factors impacting the health indicators provide root cause awareness and guidance for correction.
This predictive asset health indication can be built without the need for additional condition sensors. Process variables used for monitoring and process control can be used for asset health monitoring. The information available from electrical devices such as variable speed drives and MCCs can be particularly useful.
Building Cognitive Asset Health Management Systems
Machine learning, combined with CEP (Complex Event Processing), both available in the Quartic Platform™ can be used to build multiple machine learning agents for an asset.
By combining this intelligence with business systems information, digital twins, and creating business logic and rules with Reckon, comprehensive cognitive asset health monitoring applications are built by users in process manufacturing industries.
Contact Quartic.ai to build your first intelligent asset – yourself or with assistance from Quartic.ai; and then scale to predictive maintenance for a process unit, a plant or the entire enterprise.